Boris Hanin
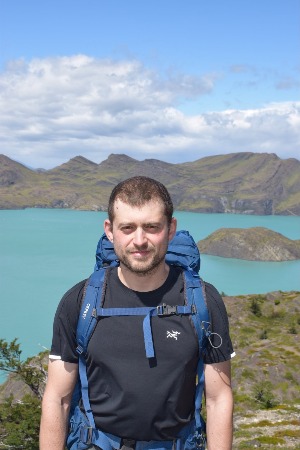
About Me
I am an Assistant Professor at Princeton ORFE working on deep learning, probability, and spectral asymptotics. Prior to Princeton, I was an Assistant Professor in Mathematics at Texas A&M, an NSF Postdoc at MIT Math, and a PhD student in Math at Northwestern, where I was supervised by Steve Zelditch.
I am also an advisor and member of the technical staff at Foundry, an incredible AI/computing startup that seeks to orchestrate the world’s compute.
Funding: I am grateful to be supported by a 2024 Sloan Fellowship in Mathematics, NSF CAREER grant DMS-2143754 and NSF grants DMS-1855684, DMS-2133806.
Please see my CV for more information.
Email: bhanin ‘at’ princeton.edu
News
- Together with Gage DeZoort, Mariangela Lisanti, Dan Marlow, and Peter Elmer, I am co-organizing at new seminar on Machine Learning in Physics. Here is the website.
- Together with Jacob Foster (Sociology, UCLA), Jessica Flack (Collective Computation Group, Santa Fe Institute), Josh Tenenbaum (Brain and Cognitive Science, MIT), Max Kleiman-Weiner (Common Sense Machines), Orit Peleg (Computer Science, Colorado), Patrick Shafto (Rutgers/IAS, Mathematics), Pranab Das (Physics, Elon), Tom Griffiths (AI & Cognitive Science, Princeton) I am organizing a semester-long program at IPAM in Fall 2024 on the Mathematics of Intelligences. This program will bring together researchers working on learning in both biological and artifical settings with the goal of identifying and making progress on mathemcial questions related to learning and intelligence. Here is the website.
- I am organizing in Summer 2024 another installment of the Princeton Machine Learning Theory Summer School website.
Research Group
Professional Service
I am an Associate Editor of
All these journals are always looking for high quality submissions on theoretical machine learning.
Short Courses
- Mathematics Aspects of Deep Learning Theory. University of Luxembourg. June 2024.
- Neural Networks and Gaussian Processes. Tor Vergata (Rome). Jan 2023. notes; Lecture 1 video, Lecture 2 video, Lecture 3 video
- Neural Networks at Large and Infinite Width (joint with Yasaman Bahri). Les Houches Summer School on Statistical Physics of Machine Learning (France). July 2022. Lecture 1 video, Lecture 2 video, Lecture Notes arXiv
Deep Learning
Preprints
- Networks of Networks: Complexity Class Principles Applied to Compound AI Systems Design, with J. Q. Davis, L. Chen, P. Bailis, I. Stoica, M. Zaharia (2024) ArXiv
- Bayesian Inference with Deep Weakly Nonlinear Networks, with A. Zlokapa (2024) ArXiv
- Are More LLM Calls All You Need? Towards Scaling Laws of Compound Inference Systems, with L. Chen, J. Q. Davis, P. Bailis, M. Zaharia, I. Stoica, and J. Zou (2024) ArXiv
- Quantitative CLTs in Deep Neural Networks, with S. Favaro, D. Marinucci, I. Nourdin, and G. Pecatti (2023) ArXiv
- Depth Dependence of μP Learning Rates in ReLU MLPs, with S. Jelassi, Z. Ji, S. Reddi, S. Bhojanapalli, and S. Kumar (2023) ArXiv
- Ridgeless Interpolation with Shallow ReLU Networks in 1D is Nearest Neighbor Curvature Extrapolation and Provably Generalizes on Lipschitz Functions (2021) ArXiv
- Approximating Continuous Functions by ReLU Nets of Minimal Width, with M. Sellke (2017) ArXiv
Journal Articles
- Random Fully Connected Neural Networks as Perturbatively Solvable Hierarchies. Journal of Machine Learning Research (2024) ArXiv
- Principles for Initialization and Architecture Selection in Graph Neural Networks with ReLU Activations, with G. DeZoort. SIAM Journal on Mathematics of Data Science (2024) ArXiv
- Bayesian Interpolation with Deep Linear Networks, with A. Zlokapa, Proceedings of the National Acacdemy of Science (2023) ArXiv
- Random Neural Networks in the Infinite Width Limit as Gaussian Processes, Annals of Applied Probability, 2023, Vol. 33, No. 6A, 4798 – 4819 ArXiv
- Non-asymptotic Results for Singular Values of Gaussian Matrix Products, with G. Paouris. GAFA (2021) ArXiv
- Products of Many Large Random Matrices and Gradients in Deep Neural Networks, with M. Nica. Communications in Mathematical Physics (2020) ArXiv
- Neural Network Approximation, with R. DeVore and G. Petrova, Acta Numerica (2020) ArXiv
- Nonlinear Approximation and (Deep) ReLU Networks, with I. Daubechies, R. DeVore, S. Foucart, and G. Petrova. Constructive Approximation (Special Issue on Deep Networks in Approximation Theory) (2019) ArXiv
- Universal Function Approximation by Deep Neural Nets with Bounded Width and ReLU Activations. Mathematics 2019, 7(10), 992 (Special Issue on Computational Mathematics, Algorithms, and Data Processing) ArXiv
Conference Articles
- Depthwise Hyperparameter Transfer in Residual Networks: Dynamics and Scaling Limit, with B. Bordelon, L. Noci, M. Li, and C. Pehlevan. ICLR 2024. ArXiv
- Principled Architecture-Aware Scaling of Hyperparameters, with W. Chen, J. Wu, and Z. Wang. ICLR 2024. Arxiv
- Maximal Initial Learning Rates in Deep ReLU Networks, with G. Iyer and D. Rolnick, ICML 2023 ArXiv
- Deep Architecture Connectivity Matters for Its Convergence: A Fine-Grained Analysis with W. Chen, W. Huang, X. Gong, Z. Wang, NeurIPS 2022 ArXiv
- Finite Depth and Width Corrections to the Neural Tangent Kernel, with M. Nica, Splotlight at ICLR 2020 ArXiv
- Deep ReLU Networks Preserve Expected Length, with R. Jeong and D. Rolnick, ICLR 2022 ArXiv
- How Data Augmentation affects Optimization for Linear Regression, with Y. Sun NeurIPS 2021 ArXiv
- Deep ReLU Networks Have Surprisingly Few Activation Patterns, with D. Rolnick, NeurIPS 2019 ArXiv
- Complexity of Linear Regions in Deep Networks, with D. Rolnick, ICML 2019 ArXiv
- Which Neural Net Architectures Give Rise to Vanishing and Exploding Gradients? NIPS 2018 ArXiv
- How to Start Training: The Effect of Initialization and Architecture, with D. Rolnick. NIPS 2018 ArXiv
Spectral Theory
Journal Articles
- Scaling Asymptotics of Spectral Wigner Functions, with S. Zelditch. Journal of Physics A (Special Edition on Claritons and the Asymptotics of Ideas: the Physics of Michael Berry) (2022) ArXiv
- Interface Asymptotics of Wigner-Weyl Distributions for the Harmonic Oscillator, with S. Zelditch. Journal d’Analyse (2022) ArXiv
- Interface Asymptotics of Eigenspace Wigner distributions for the Harmonic Oscillator, with S. Zelditch. Communications in PDE (2020) ArXiv
- Level Spacings and Nodal Sets at Infinity for Radial Perturbations of the Harmonic Oscillator, with T. Beck. International Math Research Notices, 2021. ArXiv
- Local Universality for Zeros and Critical Points of Monochromatic Random Waves, with Y. Canzani. Communication in Mathematical Physics, 2020. ArXiv
- Nodal Sets of Functions with Finite Vanishing Order, with T. Beck and S. Becker-Khan. Calculus of Variations and PDE (2018) ArXiv
- Scaling of Harmonic Oscillator Eigenfunctions and Their Nodal Sets Around the Caustic, with S. Zelditch and P. Zhou. Communications in Mathematical Physics. Vol. 350, no. 3, pp. 1147–1183, 2017. ArXiv
- C^∞ Scaling Asymptotics for the Spectral Function of the Laplacian, with Y. Canzani. The Journal of Geometric Analysis (2018) ArXiv
- Scaling Limit for the Kernel of the Spectral Projector and Remainder Estimates in the Pointwise Weyl Law, with Y. Canzani. Analysis and PDE, Vol. 8 (2015), No. 7, pp. 1707-1731. ArXiv
- High Frequency Eigenfunction Immersions and Supremum Norms of Random Waves, with Y. Canzani. Electronic Research Announcements. MS 22, no. 0, January 2015, pp. 76 - 86. ArXiv
- Nodal Sets of Random Eigenfunctions for the Isotropic Harmonic Oscillator, with S. Zelditch and P. Zhou. International Mathematics Research Notices, Vol. 2015, No. 13, pp. 4813 - 4839. ArXiv
Zeros and Critical Points of Random Polynomials
Journal Articles
- The Lemniscate Tree of a Random Polynomial, with M. Epstein and E. Lundberg. Annales Institute Henri Poincare (B), 2018. ArXiv
- Pairing of Zeros and Critical Points for Random Polynomials. Annales de l’Institut Henri Poincare (B) Probabilites et Statistiques. Volume 53, Number 3 (2017), 1498-1511. ArXiv
- Pairing of Zeros and Critical Points for Random Meromorphic Functions on Riemann Surfaces</b>. Mathematics Research Letters, Vol. 22 (2015), No. 1, pp. 111-140. ArXiv
- Correlations and Pairing Between Zeros and Critical Points of Gaussian Random Polynomials. International Math Research Notices (2015), Vol. (2), pp. 381-421. ArXiv
Other
- Contributed research to Principles of Deep Learning Theory, written by D. Roberts and S. Yaida, Cambridge University Press (2021) ArXiv
- An Intriguing Property of the Center of Mass for Points on Quadradtic Curves and Surfaces, with L. Hanin and R. Fisher. Mathematics Maganize, v. 80, No. 5, pp. 353-362, 2007.